Fundamentals of Robust Machine Learning: Handling Outliers and Anomalies in Data Science
Author: Resve A. Saleh , Sohaib Majzoub , A. K. Md. Ehsanes Saleh &
Publisher finelybook 出版社: Wiley
Edition 版本: 1st edition
Publication Date 出版日期: 2025-05-28
Language 语言: English
Print Length 页数: 416 pages
ISBN-10: 1394294379
ISBN-13: 9781394294374
Book Description
An essential guide for tackling outliers and anomalies in machine learning and data science.
In recent years, machine learning (ML) has transformed virtually every area of research and technology, becoming one of the key tools for data scientists. Robust machine learning is a new approach to handling outliers in datasets, which is an often-overlooked aspect of data science. Ignoring outliers can lead to bad business decisions, wrong medical diagnoses, reaching the wrong conclusions or incorrectly assessing feature importance, just to name a few.
Fundamentals of Robust Machine Learning offers a thorough but accessible overview of this subject by focusing on how to properly handle outliers and anomalies in datasets. There are two main approaches described in the book: using outlier-tolerant ML tools, or removing outliers before using conventional tools. Balancing theoretical foundations with practical Python code, it provides all the necessary skills to enhance the accuracy, stability and reliability of ML models.
Fundamentals of Robust Machine Learning readers will also find:
- A blend of robust statistics and machine learning principles
- Detailed discussion of a wide range of robust machine learning methodologies, from robust clustering, regression and classification, to neural networks and anomaly detection
- Python code with immediate application to data science problems
Fundamentals of Robust Machine Learning is ideal for undergraduate or graduate students in data science, machine learning, and related fields, as well as for professionals in the field looking to enhance their understanding of building models in the presence of outliers.
From the Back Cover
An essential guide for tackling outliers and anomalies in machine learning and data science.
In recent years, machine learning (ML) has transformed virtually every area of research and technology, becoming one of the key tools for data scientists. Robust machine learning is a new approach to handling outliers in datasets, which is an often-overlooked aspect of data science. Ignoring outliers can lead to bad business decisions, wrong medical diagnoses, reaching the wrong conclusions or incorrectly assessing feature importance, just to name a few.
Fundamentals of Robust Machine Learning offers a thorough but accessible overview of this subject by focusing on how to properly handle outliers and anomalies in datasets. There are two main approaches described in the book: using outlier-tolerant ML tools, or removing outliers before using conventional tools. Balancing theoretical foundations with practical Python code, it provides all the necessary skills to enhance the accuracy, stability and reliability of ML models.
Fundamentals of Robust Machine Learning readers will also find:
- A blend of robust statistics and machine learning principles
- Detailed discussion of a wide range of robust machine learning methodologies, from robust clustering, regression and classification, to neural networks and anomaly detection
- Python code with immediate application to data science problems
Fundamentals of Robust Machine Learning is ideal for undergraduate or graduate students in data science, machine learning, and related fields, as well as for professionals in the field looking to enhance their understanding of building models in the presence of outliers.
About the Author
Resve Saleh, (PhD, UC Berkeley) is a Professor Emeritus at the University of British Columbia. He worked for a decade as a professor at the University of Illinois and as a visiting professor at Stanford University. He was Founder and Chairman of Simplex Solutions, Inc., which went public in 2001. He is an IEEE Fellow and Fellow of the Canadian Academy of Engineering.
Sohaib Majzoub, (PhD, University of British Columbia) is an Associate Professor at the University of Sharjah, UAE. He also taught at the American University in Dubai, UAE and at King Saud University, KSA, and a visiting professor at Delft Technical University in The Netherlands. He is a Senior Member of the IEEE.
A. K. MD. Ehsanes Saleh, (PhD, University of Western Ontario) is a Professor Emeritus and Distinguished Professor in the School of Mathematics and Statistics, Carleton University, Ottawa, Canada. He also taught as Simon Fraser University, the University of Toronto, and Stanford University. He is a Fellow of IMS, ASA and an Honorary Member of SSC, Canada.
下载地址
相关推荐
Generative AI for Software Development: Code Generation, Error Detection, Software Testing
Motor Learning and Control: Concepts and Applications: 2024 Release
Learn Python by Doing: 100 Practical Projects for Beginners
Business Analytics: Applied Modelling and Prediction
Protecting and Mitigating Against Cyber Threats: Deploying Artificial Intelligence and Machine Learning
Artificial Intelligence (AI) in Social Research
评论 抢沙发
觉得文章有用就打赏一下
您的打赏,我们将继续给力更多优质内容
支付宝扫一扫
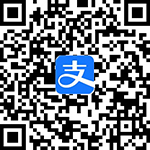
微信扫一扫
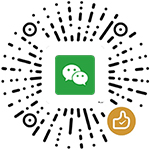