Applied Machine Learning for Data Science Practitioners
Author: Vidya Subramanian
Publisher finelybook 出版社: Wiley
Edition 版本: 1st edition
Publication Date 出版日期: 2025-05-28
Language 语言: English
Print Length 页数: 656 pages
ISBN-10: 1394155379
ISBN-13: 9781394155378
Book Description
A single-volume reference on data science techniques for evaluating and solving business problems using Applied Machine Learning (ML).
Applied Machine Learning for Data Science Practitioners offers a practical, step-by-step guide to building end-to-end ML solutions for real-world business challenges, empowering data science practitioners to make informed decisions and select the right techniques for any use case.
Unlike many data science books that focus on popular algorithms and coding, this book takes a holistic approach. It equips you with the knowledge to evaluate a range of techniques and algorithms. The book balances theoretical concepts with practical examples to illustrate key concepts, derive insights, and demonstrate applications. In addition to code snippets and reviewing output, the book provides guidance on interpreting results.
This book is an essential resource if you are looking to elevate your understanding of ML and your technical capabilities, combining theoretical and practical coding examples. A basic understanding of using data to solve business problems, high school-level math and statistics, and basic Python coding skills are assumed.
Written by a recognized data science expert, Applied Machine Learning for Data Science Practitioners covers essential topics, including:
- Data Science Fundamentals that provide you with an overview of core concepts, laying the foundation for understanding ML.
- Data Preparation covers the process of framing ML problems and preparing data and features for modeling.
- ML Problem Solving introduces you to a range of ML algorithms, including Regression, Classification, Ranking, Clustering, Patterns, Time Series, and Anomaly Detection.
- Model Optimization explores frameworks, decision trees, and ensemble methods to enhance performance and guide the selection of the most effective model.
- ML Ethics addresses ethical considerations, including fairness, accountability, transparency, and ethics.
- Model Deployment and Monitoring focuses on production deployment, performance monitoring, and adapting to model drift.
From the Back Cover
Applied Machine Learning for Data Science Practitioners
Vidya Subramanian
A single-volume reference on data science techniques for evaluating and solving business problems using Applied Machine Learning (ML)
Applied Machine Learning for Data Science Practitioners offers a practical, step-by-step guide to building end-to-end ML solutions for real-world business challenges, empowering data science practitioners to make informed decisions and select the right techniques for any use case.
Unlike many data science books that focus on popular algorithms and coding, this book takes a holistic approach. It equips you with the knowledge to evaluate a range of techniques and algorithms. The book balances theoretical concepts with practical examples to illustrate key concepts, derive insights, and demonstrate applications. In addition to code snippets and reviewing output, the book provides guidance on interpreting results.
This book is an essential resource if you are looking to elevate your understanding of ML and your technical capabilities, combining theoretical and practical coding examples. A basic understanding of using data to solve business problems, high school-level math and statistics, and basic Python coding skills are assumed.
Written by a recognized data science expert, Applied Machine Learning for Data Science Practitioners covers essential topics, including:
- Data Science Fundamentals that provide you with an overview of core concepts, laying the foundation for understanding ML.
- Data Preparation covers the process of framing ML problems and preparing data and features for modeling.
- ML Problem Solving introduces you to a range of ML algorithms, including Regression, Classification, Ranking, Clustering, Patterns, Time Series, and Anomaly Detection.
- Model Optimization explores frameworks, decision trees, and ensemble methods to enhance performance and guide the selection of the most effective model.
- ML Ethics addresses ethical considerations, including fairness, accountability, transparency, and ethics.
- Model Deployment and Monitoring focuses on production deployment, performance monitoring, and adapting to model drift.
About the Author
Vidya Subramanian is a passionate Data Science and Analytics leader, with experience leading teams at Google, Apple, and Intuit. Forbes recognized her as one of the “8 Female Analytics Experts From The Fortune 500.” She authored Adobe Analytics with SiteCatalyst (Adobe Press) and McGraw-Hill’s PMP Certification Mathematics (McGraw Hill). Vidya holds Master’s degrees from Virginia Tech and Somaiya Institute of Management (India) and currently leads Data Science and Analytics for Google Play.
下载地址
相关推荐
Unlocking Python: A Comprehensive Guide for Beginners
Getting Started with .NET Aspire: Build Cloud-Native and Distributed Applications with Ease
Securing Our Future: A Science-Based Approach to Global Threats
6G Cyber Security Resilience: Trends and Challenges
Procedural Content Generation Via Machine Learning: An Overview, 2nd Edition
Embedded Microprocessor System Design using FPGAs 2nd Edition
评论 抢沙发
觉得文章有用就打赏一下
您的打赏,我们将继续给力更多优质内容
支付宝扫一扫
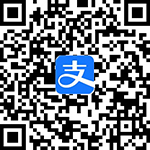
微信扫一扫
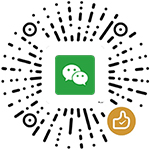