Time Series Forecasting Using Generative AI: Leveraging AI for Precision Forecasting
Author: Banglore Vijay Kumar Vishwas , Sri Ram Macharla
Publisher finelybook 出版社: Apress
Edition 版本: First Edition
Publication Date 出版日期: 2025-03-25
Language 语言: English
Print Length 页数: 231 pages
ISBN-10:B0DQC953BV
ISBN-13: 9798868812750
Book Description
“Time Series Forecasting Using Generative AI introduces readers to Generative Artificial Intelligence (Gen AI) in time series analysis, offering an essential exploration of cutting-edge forecasting methodologies.”
The book covers a wide range of topics, starting with an overview of Generative AI, where readers gain insights into the history and fundamentals of Gen AI with a brief introduction to large language models. The subsequent chapter explains practical applications, guiding readers through the implementation of diverse neural network architectures for time series analysis such as Multi-Layer Perceptrons (MLP), WaveNet, Temporal Convolutional Network (TCN), Bidirectional Temporal Convolutional Network (BiTCN), Recurrent Neural Networks (RNN), Long Short-Term Memory (LSTM), Deep AutoRegressive(DeepAR), and Neural Basis Expansion Analysis(NBEATS) using modern tools.
Building on this foundation, the book introduces the power of Transformer architecture, exploring its variants such as Vanilla Transformers, Inverted Transformer (iTransformer), DLinear, NLinear, and Patch Time Series Transformer (PatchTST). Finally, The book delves into foundation models such as Time-LLM, Chronos, TimeGPT, Moirai, and TimesFM enabling readers to implement sophisticated forecasting models tailored to their specific needs.
This book empowers readers with the knowledge and skills needed to leverage Gen AI for accurate and efficient time series forecasting. By providing a detailed exploration of advanced forecasting models and methodologies, this book enables practitioners to make informed decisions and drive business growth through data-driven insights.
● Understand the core history and applications of Gen AI and its potential to revolutionize time series forecasting.
● Learn to implement different neural network architectures such as MLP, WaveNet, TCN, BiTCN, RNN, LSTM, DeepAR, and NBEATS for time series forecasting.
● Discover the potential of Transformer architecture and its variants, such as Vanilla Transformers, iTransformer, DLinear, NLinear, and PatchTST, for time series forecasting.
● Explore complex foundation models like Time-LLM, Chronos, TimeGPT, Moirai, and TimesFM.
● Gain practical knowledge on how to apply Gen AI techniques to real-world time series forecasting challenges and make data-driven decisions.
Who this book is for:
Data Scientists, Machine learning engineers, Business Aanalysts, Statisticians, Economists, Financial Analysts, Operations Research Analysts, Data Analysts, Students.
From the Back Cover
“Time Series Forecasting Using Generative AI introduces readers to Generative Artificial Intelligence (Gen AI) in time series analysis, offering an essential exploration of cutting-edge forecasting methodologies.”
The book covers a wide range of topics, starting with an overview of Generative AI, where readers gain insights into the history and fundamentals of Gen AI with a brief introduction to large language models. The subsequent chapter explains practical applications, guiding readers through the implementation of diverse neural network architectures for time series analysis such as Multi-Layer Perceptrons (MLP), WaveNet, Temporal Convolutional Network (TCN), Bidirectional Temporal Convolutional Network (BiTCN), Recurrent Neural Networks (RNN), Long Short-Term Memory (LSTM), Deep AutoRegressive(DeepAR), and Neural Basis Expansion Analysis(NBEATS) using modern tools.
Building on this foundation, the book introduces the power of Transformer architecture, exploring its variants such as Vanilla Transformers, Inverted Transformer (iTransformer), DLinear, NLinear, and Patch Time Series Transformer (PatchTST). Finally, The book delves into foundation models such as Time-LLM, Chronos, TimeGPT, Moirai, and TimesFM enabling readers to implement sophisticated forecasting models tailored to their specific needs.
This book empowers readers with the knowledge and skills needed to leverage Gen AI for accurate and efficient time series forecasting. By providing a detailed exploration of advanced forecasting models and methodologies, this book enables practitioners to make informed decisions and drive business growth through data-driven insights.
● Understand the core history and applications of Gen AI and its potential to revolutionize time series forecasting.
● Learn to implement different neural network architectures such as MLP, WaveNet, TCN, BiTCN, RNN, LSTM, DeepAR, and NBEATS for time series forecasting.
● Discover the potential of Transformer architecture and its variants, such as Vanilla Transformers, iTransformer, DLinear, NLinear, and PatchTST, for time series forecasting.
● Explore complex foundation models like Time-LLM, Chronos, TimeGPT, Moirai, and TimesFM.
● Gain practical knowledge on how to apply Gen AI techniques to real-world time series forecasting challenges and make data-driven decisions.
About the Author
Bangalore Vijay Kumar Vishwas (B.V. Vishwas) is a seasoned Principal Data Scientist and AI researcher with over 11 years of experience in the IT industry. Currently based in San Diego, California, he works at NTT DATA. Vishwas holds a Master of Technology in Software Engineering from Birla Institute of Technology & Science, Pilani, India. He specializes in developing innovative solutions for large enterprises, with expertise in Machine Learning, Deep Learning, Time Series Forecasting, Natural Language Processing, Reinforcement Learning, and Generative AI. He is also the author of Hands-On Time Series Analysis with Python: From Basics to Bleeding-Edge Techniques, published by Apress.
Sri Ram Macharla, is a consultant and architect in the areas of AI and ML with over 19 years of experience in IT. He holds an M.Tech from BITS Pilani and has experience working with clients in domains such as finance, retail, life sciences, defense, and manufacturing. Additionally, he has worked as a mentor, corporate trainer, and guest faculty teaching AI and ML. He has papers published and works as a reviewer with leading journals and publishers. He is passionate about mathematical modeling and applying AI for social good.
下载地址
相关推荐
Unlocking Python: A Comprehensive Guide for Beginners
Getting Started with .NET Aspire: Build Cloud-Native and Distributed Applications with Ease
Felix Klein: The Erlangen Program
Securing Our Future: A Science-Based Approach to Global Threats
6G Cyber Security Resilience: Trends and Challenges
Procedural Content Generation Via Machine Learning: An Overview, 2nd Edition
评论 抢沙发
觉得文章有用就打赏一下
您的打赏,我们将继续给力更多优质内容
支付宝扫一扫
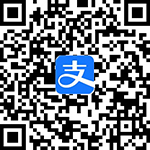
微信扫一扫
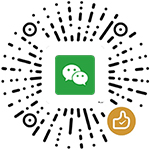